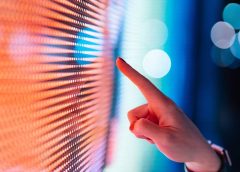
How Fashion Retailers Use AI to Optimise E-Commerce and Consumer Experience
[ad_1]
Launched in 2015 by co-founders Purva Gupta and Sowmiya Narayanan, AI-powered platform Lily AI was founded to help accurately connect a retailer’s customer network with the relevant products they’re seeking online, at scale.
By utilising AI to create a robust product taxonomy of product attributes, the programme is designed to give consumers a more bespoke e-commerce experience through improved on-site search conversion and personalised product discovery journeys. Today, it has grown in size and scope and counts fashion retailers and brands as varied as Macy’s, Joor, The Gap, Bloomingdale’s, Vans and ThredUp among its clients.
While technology has already revolutionised the way that global fashion companies do business, the Covid-19 pandemic — and subsequent shifting shopping behaviours — affirmed how critical technological innovation is to success and continued growth.
Indeed, The Business of Fashion’s State of Fashion: Technology report, a special edition of BoF’s flagship report, produced in partnership with McKinsey & Co., identifies “hyper-personalisation” as one of its five technology-driven imperatives for the fashion industry, citing opportunities for executives to harness Big Data and AI to provide personalised, one-to-one experiences that build long-term loyalty. In fact, more than 60 percent of fashion executives believe creating integrated digital processes throughout their organisations will be among their top five areas for digitisation as they look ahead to 2025.
:quality(70)/cloudfront-eu-central-1.images.arcpublishing.com/businessoffashion/EDVELOFLPZANJMGFSV2HWPYTGU.png)
Now, BoF sits down with Lily AI co-founder Purva Gupta to gain insight into Lily AI’s use-cases, the benefits of personalising shopper journeys through technology, and the relationship between Artificial Intelligence and Customer Centricity.
What insights can Lily AI share around evolving shopper behaviour?
The pandemic led to huge amounts of digital escalation, which essentially led to skyrocketing expectations of shoppers. Post-pandemic, even though the online/offline split may have returned in some ways, the expectation of the shopper has just completely changed. We now operate in a world where players like Amazon are completely changing the way that consumers are experiencing products — from speed to accuracy and [breadth] of product mix. As a result, customer demand for a frictionless online experience is unrelenting.
Fundamentally, the entire retail stack needs to absorb what we call the language of the customer. Let me give you an example. If a consumer is looking for a loose dress, there are 50 other ways a shopper might search for it. They could type in a sun dress, nap dress or a swing dress — there are so many different ways of searching for the same thing. Retailers aren’t accounting for this difference in language and [terminology].
How does Lily AI’s technology work?
AI is when a machine mimics what humans can do, but at scale. Your AI is only as good as your training models and your training data, so we made sure we had great training data that our domain experts collected in-house.
If a consumer is looking for a loose dress, there are 50 other ways a shopper might search for it. Retailers aren’t accounting for this difference in language and [terminology].
Our AI is able to ingest any type of product catalogue from a brand or retailer. It can then extract extensive, minute attributes out of those images and text in the product catalogue. Then, through our platform, our customer is able to map those attributes to their internal workflows to make sure that it’s aligned with how internally all the different types of operations are using that data. They can customise it; they can do whatever they want to do with respect to making sure that it’s aligned.
How are its use-cases evolving?
Right now, as of this moment, there are about half a dozen applications that Lily AI is currently working on with our brands and retailers. There are another half a dozen meaningful applications that these brands and retailers have already shared that they’d like us to explore together. Now, it’s a question of discovering how many different applications and integrations can be conceived.
Once we have all these deep attributes via the Lily platform, we are able to send them to different destination systems in the retail stack, from search engines to a recommender system and even a demand forecasting application. Those are all different types of applications that are consuming this rich product attribute data.
It’s [critical] to know that just giving these attributes to retailers is not enough. It’s about taking this information to the right destination systems and closing the loop, so that retailers are able to see the ROI from all the different applications that they could use this data in.
What examples of best-in-class strategy can you share for other retailers to be guided by?
One of the retailers that we work with saw their relevant search results increase thirtyfold. Results like that have the power to be transformative, because the end consumer is going to notice an improvement. That’s really the best compliment we can receive — when not just retailers, but the end customers directly are noticing the improvements.
Just giving these attributes to retailers is not enough. It’s about taking this information to the right destination systems and closing the loop.
Thanks to those improved search results, you’re also getting 30 times better business results. For this retailer, that translated into at least $20 million in incremental revenue for the company, just from search alone.
How can brands use Artificial Intelligence to become more customer-centric?
The consumer’s language is missing overall in the retail game today. The amount of data that is getting used upstream across decision-making is so small. This simple update can help to keep the customer at the centre of your business and lead to significant lifts in revenue. Let’s say that your consumer is looking for a statement blazer. Attributes for a statement blazer may not be easily manually applied by merchants today. If retailers are attributing products purely with legacy, out-of-the-box vernacular, it could mean they are missing out on important consumer context, thus giving customers a poor search or recommendation experience.
If you look at behavioural data of shoppers — how they interact with an online store, where they click, what they buy, what they return — all of that behavioural data has been used for the last 20-plus years in all sorts of experiences. However, when it comes to product data, this is truly low-hanging fruit that has yet to be fully explored by retailers. It’s a critical way for retailers to decipher deeper consumer behaviour, and in turn, become more customer-centric.
Mid-term, what excites you most about merging AI and e-commerce capabilities?
What I find so exciting is that, ultimately, this isn’t rocket science. What is happening in this space is so intuitive. It’s the language of the consumer — the way a shopper looks for things, the way they analyse — that has been missing from the entire retail [ecosystem]. For me, this could fundamentally transform how retail works, as businesses will know so much more about their customer, both online and offline. There are lots of different types of narratives around AI and how it does or does not work. I’m so glad that we have a story here, where we have found something so fundamentally intuitive that retail really needs. We’ve been able to solve image recognition — a traditionally hard technology problem.
The second element I am excited about is the closed loop that AI can provide within retail. You can build really good technological solutions, but if you can’t put them to use and deliver ROI, close the loop for retailers and for the end consumer, then you’re missing a piece of the puzzle.
This is a sponsored feature paid for by Lily AI as part of a BoF partnership.
[ad_2]
Source link